Visual boundary segmentation: Psychophysics
Segmenting luminance-defined texture boundaries
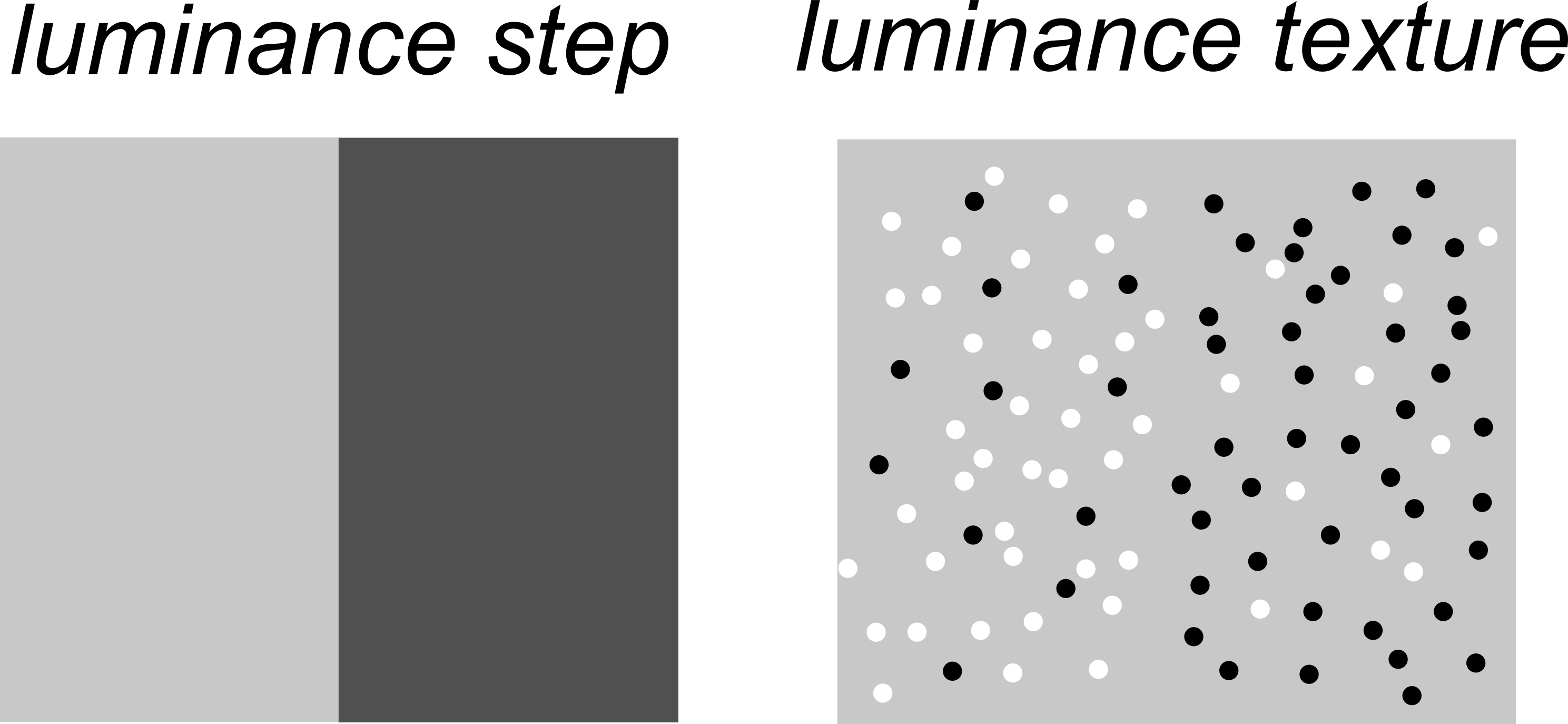
Often two adjacent image regions belonging to different surfaces have a clear difference in regional luminance, without giving rise to a sharp step edge. However, it has not been systematically investigated whether these texture-defined luminance boundaries are detected using the same mechansism as luminance step boundaries. We are conducting a series of studies to address this issue carefully and to gain insight into possible neural mechanisms. This work is a collaboration with Curtis Baker at McGill Univeristy.
Combination of first- and second-order cues for boundary segmentation
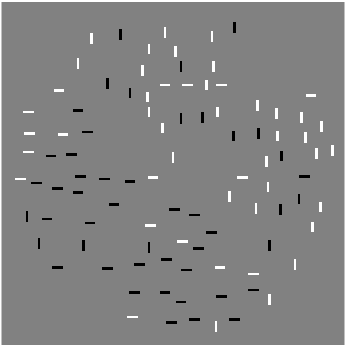
Natural boundaries are defined by a number of cues, including differences in luminance, color and texture (DiMattina, Fox, & Lewicki 2012), all of which are integrated in perceptual tasks. However, the exact mathematical rules for cue combination for boundary segmentation, when boundaries are defined by first-order and second-order cues, remain little explored. We are working on studying how cues are combined for boundary segmentation using artifical boundaries combining luminance, texture and color cues, in the hope of better understanding the mechansisms of natural boundary segmentation. This work is a collaboration with Curtis Baker and Fred Kingdom at McGill Univeristy.
Behavioral decoding of deep neural networks
Boundary segmentation and shadow detection
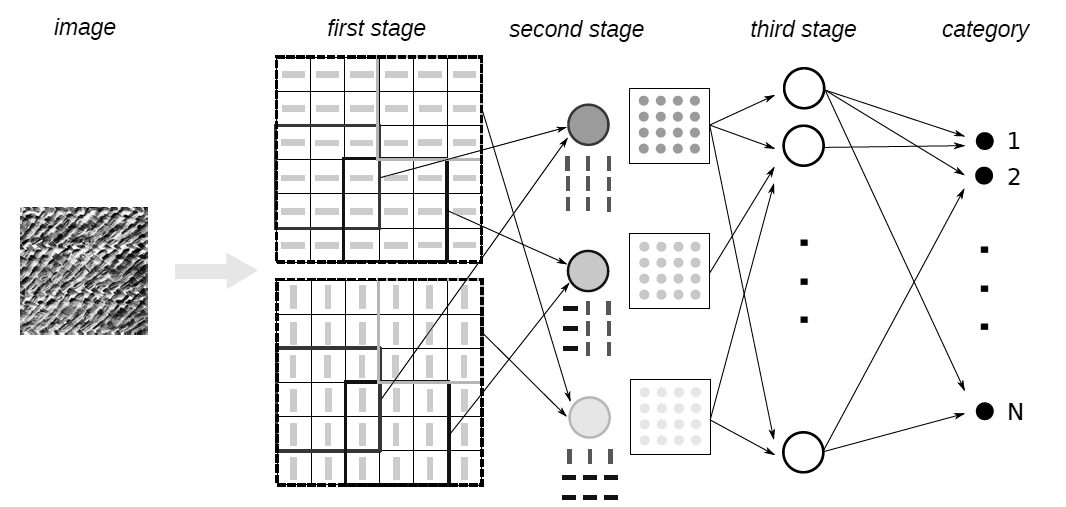
Previous computational work has shown that deep neural networks trained on object recognition tasks learn intermediate layer representations with nonlinear "receptive field" properties similar to those observed in visual areas V2 and V4. I am currently extending this line of inquiry by analyzing the performance of such networks on mid-level vision tasks like boundary segmentation and shadow detection, and training behavioral decoding models based on these networks to predict human psychophysical observations.